Very few people work alone in the so-called knowledge economy. Even a lowly freelance science writer has a network of editors, publishers and other associates on which they rely to get their words out to an audience. The point is even more apparent in the world of research where often vast teams of experts must pull together to generate a result. Just look at the author list on almost any genomics or post-genomics research paper from the past decade or so to see just how true that is.
Neil Rubens, Mikko Vilenius, and Toshio Okamoto of the Graduate School of Information Systems, at the University of Electro-Communications, in Tokyo, Japan, and Dain Kaplan of the Department of Computer Science, Tokyo Institute of Technology, certainly recognise this fact. They have, however, spotted the obvious flaw in collaborative working – how to find the right “expert” for the task in hand.
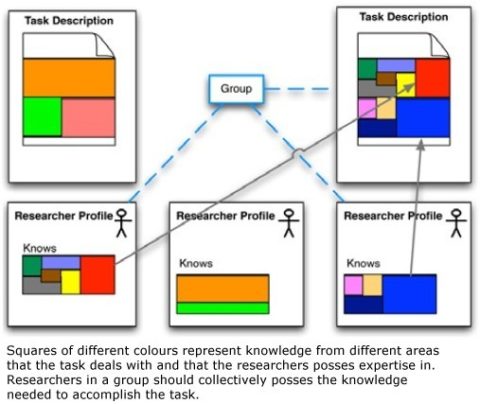
Writing in the International Journal of Knowledge and Web Intelligence, they have come up with a possible solution to this perennial problem. They explain that research-oriented tasks are increasingly complex in nature and require more sophisticated networks of experts. In the past, finding a single expert for a task or pulling together an expert group was done in an almost ad hoc manner. They have now devised a method that could automate the process and allow the best experts to be pooled to complete a given task. “Simply speaking, we are connecting tasks and people,” the team explains. “Our goal is to predict who has the needed expertise to accomplish the task at hand.”
The researchers have created a formula that can pull in the most suitable experts from a database and that weights each expert according to various criteria for the specific task, their proof of principle was to pool experts to write an academic paper. They then “train” their expert finding model using a random selection of 1000 published papers. The team explains the procedure as follows:
“For each paper we fix the size of the candidate author pool at 100, containing the real authors, and then randomly selected ones. We then create 20 sets of authors randomly selected from the pool along with one set of actual authors (all sets are of equal size). Data from one half of the randomly selected papers is used to train the model. The other half is used to evaluate the model.” They add that, “We have performed several different training/testing data splits and obtained similar results.”
Presumably, once proven more widely the model could be incorporated into software that might be used by recruitment agencies, headhunters, and individuals or groups hoping to pull together experts for particular projects and job vacancies.
Neil Rubens, Mikko Vilenius, Toshio Okamoto, & Dain Kaplan (2010). CAFE: Collaboration Aimed at Finding Experts Int. J. Knowledge and Web Intelligence, 1 (3/4), 169-186